Introduction
We are being inundated with a deluge of genomic data [1]. Some reasons include declining sequencing and storage costs and increasing computing power. Sequencing technology is advancing faster than Moore’s Law [2], [3]. This astounding growth is due in part to the open-data and open-source mentality of researchers.
Nearly universal agreements among scientists have fostered this mentality. One such agreement is the Fort Lauderdale Agreement in 2003, which is a renewal of the Bermuda Principles of 1996. Researchers called for rapid publication of large sequence datasets. The Agreement established a tripartite system of responsibility to ensure that this community sharing system continues to flourish. These three parties are funding agencies, resource producers, and resource users [4].
Now that we have access to vast amounts of genomic data, it’s only natural to investigate whether individuals could be identified by genomic analysis. Indeed, research by Homer et al. and Lin et al. showed that individuals could be identified from genomic data alone. Lin et al. showed that only 75 SNPs would be sufficient to narrow it down drastically [5]. Homer et al. showed that composite statistics such as allele frequency are not sufficient to mask identity within genome-wide association studies [6].
It is important to note that Homer et al.’s findings were tempered somewhat by Braun et al.’s work. They found that Homer et al.’s methods had a high sensitivity (i.e. correct classification of true positives) but also had a low specificity (i.e. correct classification of true negatives). GWAS samples are drawn from a tiny portion of the population. Suppose a person with malevolent intentions possessed a genome with a 33% probability of being part of a GWAS dataset. That malefactor would need a sensitivity of 99% and a specificity of 95% to have 90% confidence in an identification. Homer et al.’s method does not perform up to these requirements [7].
Nevertheless, these findings resounded through the scientific community. Scientists worried that there would be burdensome regulations imposed by the government, constraining them from doing research. They also worried that samples posted online in massive databases would violate people’s privacies. Another point of contention was that as technology progresses, the ability to identify individuals based on publicly available genetic data would improve. This would retroactively invalidate donors’ informed consents. Yet another concern was that open data access would soon become closed, at odds with the open-data/open-source movement [8]. These concerns, among others, are discussed in this paper. In particular, (de)identification techniques and improved consent procedures are examined.
Identification Techniques
There are several identification techniques that can discern an individual’s genome out of aggregate data and publicly available data. These techniques rely on a variety of principles such as linkage disequilibrium (LD) and single nucleotide polymorphisms (SNPs). It is conceivable that malefactors and actors with an interest in identification could use these techniques to put a name to a unique genome. For instance, an insurance company could covertly identify an individual’s genome. If that individual’s genome shows a predisposition to costly diseases, the company could deny coverage, although not ostensibly for that reason. Another possibility is that there could be a “Facebook” of genomic data. Identified and unwitting individuals could unknowingly have their data available online for perusal. Potential mates could use this database to vet their prospective partners. The Genetic Information Nondiscrimination Act (GINA) is supposed to prevent such abuse, but as discussed before, GINA is not a panacea.
These are just hypothetical situations. One prominent real-life example is the controversy surrounding the publication of Dr. James Watson’s genome. Watson, a co-discoverer of DNA’s structure, requested that the sequences surrounding his ApoE gene be redacted [9]. APOE codes for apolipoprotein E. Some variants of ApoE such as the E4 variant is strongly associated with late-onset Alzheimer’s disease [10]. Analysis using LD can reveal what was redacted. Although the authors of [9] kindly don’t divulge Watson’s ApoE status, they used the genome of Dr. J Craig Venter to illustrate their point that LD is a powerful technique to impute and infer redacted regions. Indeed, they were able to identify Venter’s ApoE genotype as high-risk with probability of 99.2% [9].
The imputation of Watson’s and Venter’s ApoE genotype utilized LD analysis, one of many techniques to infer redacted regions. LD can be used to identify missing regions. Now what about techniques that can put a name to anonymized genomes? A study by Homer et al. investigated the possibility of resolving an individual’s genomic DNA out of a complex mixture of several people’s genomic DNA. They used a method called high-density single nucleotide polymorphism (SNP) genotyping microarrays combined with advanced statistical analysis. Their simulations showed that 10,000 to 50,000 SNPs can resolve mixtures to which the genomic DNA of an individual contributes at a minimum of 0.1%. The number of SNPs can be even less if the SNPs are carefully selected to minimize variation across populations.
Homer et al. also did experimental validation. In mixtures of 40+ people, all individuals were identified with no false positives. Also, the authors could resolve individuals by using their parents’ genotypes, albeit with a higher rate of false positives. Finally, the group was able to resolve an individual contributing less than 1% of genomic DNA to a mixture [6]. These methods, when used on publicly available genome-wide association studies (GWAS), can tease out a single individual’s contribution to a mixture. This has enormous implications on making genomic data publicly available.
Homer et al.’s findings, however, were met with some skepticism. Braun et al. investigated the limitations of Homer et al.’s methods. They found that Homer et al.’s method had a quite high sensitivity, but a lower specificity than predicted. Their findings temper Homer et al.’s findings somewhat. This is not to say that the methods pioneered by Homer et al. are useless for individual identification, but rather that it is harder than previously thought. If a malefactor (i.e. a person who is interested in identification) has prior knowledge that an individual is contained within a mixture with at least 33% probability, the method still needs to have a sensitivity of 99% and a specificity of 95%. Braun et al. closes by noting that the methods proposed by [6] fail to “function as a tool to positively identify the presence of a specific individual’s DNA in a finite genetic sample” [7].
Butler describes the history of short tandem repeats (STRs) becoming integral to human identity testing [11]. STRs were first realized as a boon for forensics by Edwards et al. [12], [13]. STRs have been mainly used for two purposes: criminal forensic testing and paternity testing. The FBI curates a Combined DNA Index System (CODIS) database that utilizes 13 genetic markers. CODIS in conjunction with the U.S. National DNA Database (NDNAD) have proved useful in forensics. Furthermore, as these curated databases continue to grow, more genomes can be matched against these original entries. If a person’s blood relative has sequences stored in CODIS or NDNAD, it is likely that the person can also be identified by virtue of similar DNA [11].
Building upon the idea of using STRs to identify humans, scientists took advantage of the fact that STRs located on the Y chromosome (Y-STRs) is often correlated with surnames since surnames are patrilineal [14]. Adoptees seeking the identity of their biological fathers were able to leverage genetic genealogy databases along with Y-STRs [15]. Lunshof et al. were the first to propose that the freely available genetic genealogy databases could be exploited to fully identify individuals participating in sequencing projects [16]. Indeed, Gitschier was the first to connect surnames to Utahn participants’ genomes in genealogical databases [17]. Gymrek et al. built upon this work with the goal of surname identification in a more general population. They used the freely available genealogical databases Ysearch (www.ysearch.org) and SMGF (www.smgf.org). Their analysis showed a success rate of approximately 12% of identifying surnames of white males using Y-STRs. Gymrek et al. cautions that obfuscating or redacting Y-STRs alone is not sufficient to protect against identification attacks. This is due to the possibility of imputing and inferring Y-STRs based on SNPs elsewhere on the Y chromosome using LD. This would bypass Y-STR masking [18].
Using SNPs to assist genome identification is a common tactic. Lin et al.’s work is no exception. They found that “only 30 to 80 statistically independent SNP positions” is sufficient to identify an individual. Figure 1, taken from Lin et al.., shows the relationship between the number of independent SNPs and privacy guarantee. Generally, as independent SNPs approach ~75 SNPs, the privacy guarantee drops to nearly zero. To put this in context, chromosome 21 has 4,563 independent SNPs [5].
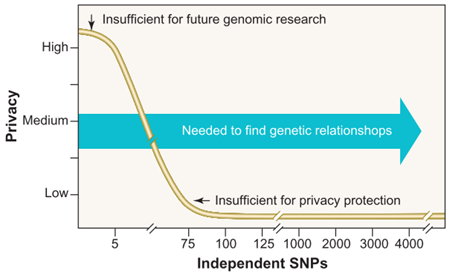
Lowrance et al. [19] summarizes three categories of identification techniques. The first is matching against a reference genotype. Work by Lin et al. [5], Butler [11], and Homer et al. [6] fall in this category. Reference genotypes are easily found in online databases such as CODIS, genealogical services, and federally-funded sequencing projects.
The second category is linking to nongenetic databases. This involves a bit more detective work. Genomic data could be linked to non-genetic data such as the usual demographics (i.e. gender, age, ethnicity, etc).
The third category is profiling from genomic data. It is possible to infer phenotypical attributes from genomic data. Currently, it is trivial to learn the gender, blood type, skin pigmentation, presence of disorders from genomes alone. Work is being done to improve prediction of height, weight, hair color, eye color, and even facial features [20]–[22].
De-Identification Techniques
Identification techniques are improving and widespread. These techniques, while a great boon to research and forensics, are ominous with respect to privacy. Efforts by several groups are being made to find robust methods to de-identify genomes so that they are not susceptible to (re)identification attacks. This section will explore these efforts.
Before diving into genomic de-identification, it is important to note that great strides have been made in electronic health record (EHR) de-identification. Meystre et al. notes that over 200 publications since 1995 have been made regarding EHR de-identification. Keeping EHRs anonymous when part of online datasets is a critical part of the battle to protect identities of genome donors. Meystre et al. also makes an important distinction between anonymization and de-identification. De-identification is merely the removal of any identifying attributes from the record itself. Anonymization is the guarantee that the record cannot be linked back to an individual through (in)direct means. This distinction is important to keep in mind as genomic information could be easily be de-identified, but rather difficult to anonymize [23].
Malin evaluates a variety of approaches to protect privacy of genomic data. There are four systems of protection. The first is de-identification (DEID). DEID involves the classification of attributes into explicit identifiers (e.g. full name, Social Security number), quasi-identifiers (e.g. date of birth, zip code), and non-identifiers. When sharing data, data holders are responsible for erasing explicit identifiers and generalizing quasi-identifiers from metadata of genomic information.
The second system of privacy protection is denominalization (DENOM). This is similar to DEID, except that DENOM involves the stripping of familial information. Attributes such as family, relation, marriage, sibling, and so on, are removed from metadata.
The third system is trusted third parties. This was introduced by deCode Genetics Inc.. This involves the use of third parties, who are able to de/encrypt data. Thus, these third parties hold a great deal of responsibility, which may not be ideal. The fourth system, semitrusted third parties, remedies that. In a semitrusted system, third parties are only empowered to transmit encrypted data. De/encryption then occurs on the researchers’ end [24].
Lowrance and Collins outline a few additional strategies to de-identify genomic data. When genomic data is released or published, it is wise to limit the proportion of the genome that is publicly available. Sequence traces, variations, and minimal phenotypic data are released. That way, malefactors are unable to see the full genome for identification attack. However, the proportion of the genome that is released can be problematic. Lowrance and Collins note that it can be difficult to know precisely which segments are “safe” to release, especially as re-identification techniques advance. A segment that has strong linkage disequilibrium or rare variants would be inadvisable to publish. A segment with relatively few interesting features would be safe to publish, but would not be beneficial to future researchers. There is a tension between releasing too much – assisting malefactors – and releasing too little – impeding research.
Another approach to de-identification outlined by Lowrance and Collins is to statistically degrade the data by using IUPAC nucleotide nomenclature. For instance, purines (adenine and guanine) can be represented by R and pyrimidines (cytosine and thymine/uracil) can be represented by Y. So, a sequence such as GATTACA could be represented by RRYYRYR. However, RRYYRYR could just as easily refer to AGCCGTG [25]. This approach is especially detrimental to future researchers, since the ambiguity could mask the presence of a healthy variant or a pathogenic variant. Increasing ambiguity of the data decreases its usefulness to researchers.
The approach most commonly used by health researchers is to separate identifiers and the data. To re-associate identifiers and data, a key is required. This key can be entrusted to trusted parties and only handed out on a case-by-case basis. However, the data itself remains untouched so other approaches to de-identify data is required as well [19]. A problem with this approach is that keys are often stored together. If there is a breach, then the keys are divulged, resulting in a wholesale reidentification of many individuals.
Re-Identification
There is ongoing research focusing on re-identification of genomic data using nothing but de-identified data. Malin describes four techniques to do this. First, genealogical information packaged with the data can be used to reveal family structure of the donor. This structure can be confirmed by using freely available genealogical records, which then affixes a name to the de-identified donor’s genomic data.
Another attack is genotype-phenotype inference. A genotype may show that the donor has a specific phenotype, which then can be matched with clinical records. Malin’s experiments found that age of onset of simple Mendelian disease (±3 years) can be inferred from genomic data. That age of onset then can be used as a cross-reference with clinical data. This type of attack benefits from advances in research since it is becoming easier to predict a phenotype from a genotype. Eventually, craniofacial structure could even be inferred from complete genomic data.
Trail re-identification is an attack strategy that uses location to match genomic data to identity. The essence of this method is to match the location of names with the location of genomic data. For example, if John was known to be part of Anywhere Hospital’s clinical trial and a genome was released by Anywhere Hospital, it is possible to use Boolean inference to deduce that the de-identified genome is John’s. Methodology and experiments of these attack strategies are described in detail in [24].
In a ground-breaking study of re-identification, Malin and Sweeney introduce several algorithms that can link genomic data to named individuals [26]. These algorithms are collectively called re-identification of data in trails (REIDIT). REIDIT algorithms exploit publicly available records of patient-location visit patterns. These algorithms utilize relational database theory (invented by Codd [27]). Malin and Sweeney’s results are best summarized by Figure 2, below. The fewer individuals in a study released by a hospital, the greater the chance of re-identification. This correlation occurs because there are fewer inferences to make. This has implications for protecting privacy: dilute data releases by including more individuals.
Data Access Control
To prevent re-identification attacks and to protect patient privacy, several measures concerning data access control have been developed.
Habegger et al. developed a compact data summary format called the Mapped Read Format (MRF). Essentially, the MRF file summarizes information about short and long alignments, while protecting the actual sequence data. Aspects of the alignments can then be calculated without access to the genomic data. The MRF and genomic information are decoupled, protecting patients/donors [28].
Lowrance and Collins address data access issues by outlining three measures that reduce the risk of identification. The first of these calls for stronger terms of agreements. These contracts can be enforced legally, enumerating limitations and stipulations about how and why the data can be used. For example, an agreement might specify that release is conditional on total de-identification or accessible only to authorized, named researchers. These agreements are approved by Institutional Research Boards. The issue with this approach is that it depends on the good faith of everyone involved with the research project. Criminal malefactors aren’t going to care about such contracts.
Another measure is to increase oversight of data-release decisions. Before a curator of genomic data can publicly release genomic data, committees review the data and purposes before approving release. This measure is vulnerable to improvements in re-identification techniques. What was not possible to identify five years ago could now be identify nowadays.
An extreme measure is to quarantine data to enclaves (encrypted servers) where only authorized researchers can view and manipulate data. This measure should only be used as a last resort because it can impede access by peer reviewers [19].
Greenbaum and Gerstein propose the increased use of cloud computing to manage access to data. Here, sequence data is no longer kept locally. Instead, data is stored off-site within secure servers. Computing power is also supplied by these servers. Authorized researchers then need not worry about data access or having enough computing power to perform the calculations they wish to perform. Once researchers have performed analysis on the data, these anonymized and summarized results are allowed out of the cloud.
By centralizing data access control and computing power, the burden on researchers is allayed somewhat. Furthermore, cloud access requires, at the very least, a username and a password. This would provide strong surveillance of accession by researchers, promoting patient/donor privacy at the expense of researchers’ privacy. Greenbaum and Gerstein point out some pitfalls of this approach: data security and legal considerations. Centralizing data makes it much more appealing as a treasure trove to hackers and malefactors. Also, since the servers may be in a different state or country than the researcher, experiments may be subject to multiple jurisdictions. That could be headache-inducing for researchers. Nevertheless, cloud computing seems to be a promising solution for data access control [29].
Another approach to protect patient/donor privacy is to randomly censor SNPs in sequence data, as mentioned by Lin et al.. However, it is tricky to find an acceptable fraction of SNPs to censor. Altering too many SNPs would hinder researchers, while altering too few SNPs would leave the door open for malefactors.
Lin et al. also mentions the use of binning SNPs as a protection measure. Essentially, SNPs near to each other would be binned together. However, the authors reject SNP binning as a useful protection, since it would overwhelmingly obfuscate useful data. Lin et al. closes by reinforcing the idea of cloud computing as an effective approach to data access control [5].
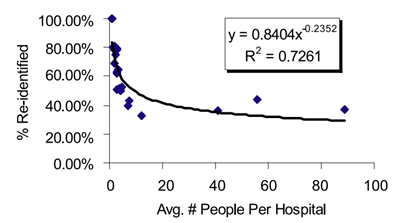
Informed Consent
It is not enough to control data access. It is also important to ensure that patients/donors are aware of and consent to ongoing experiments that use their DNA, profiles, and data. Simply having patients/donors sign documents is not enough. There is a need to improve informed consent procedures. Furthermore, since new technologies and approaches may develop, a process needs to be designed that accommodates the changing landscape of research. Concepts such as informed consent and continuous renewals of these agreements are explored in this section.
A company – 23andMe – published the first genome-wide association study (GWAS) in June 2010 [30]. The study is first to use self-reported information provided by Internet customers of 23andMe when they purchased genotyping services. The impending publication of this study concerned the editors at PLoS Genetics about consent procedures.
Participants (customers) of 23andMe’s study were given detailed consent documents, disclaiming that 23andMe’s services are not intended to serve as diagnostic tools or as medical advice. Also, the consent documents state that before sharing individual-specific data with collaborators and third parties, additional individual consent would be sought. While this demonstrates appropriate use of additional consent in new and changing circumstances, the editors took issue with the technical nature of the documents, ambiguity of what data will be published or shared, and legality.
The editors debated whether to require consent of the 3,000+ participants in the study, considering this new publication. They decided against this requirement, due to the onerous burden on researchers/lawyers to rewrite consent documents and to re-obtain consent from participants [31].
The prominent genomics rights activist, George Church, suggests the use of a “creative commons universal waiver”. Patients/donors consent to full and continuing release only with tested 100% understanding. Tests would ensure that consent is truly informed [32].
Austin et al. makes a distinction between active and presumed consent. The latter being when participation is “opt-out”. GWAS studies such as 23andMe’s study straddle the line between active and presumed consent [30]. Presumed consent is more permissible when it also has the permission of the community. There is disagreement on the process of obtaining community consent. What fraction of the community is sufficient? How are representatives selected? The difficulty of obtaining honest community consent makes it an unrealistic approach [33].
Sometimes studies involving human sequences may not even require informed consent. Once the data and identifiers have been sufficiently decoupled, further experiments using the data no longer deal with human subjects, obviating the need for consent. Work by Malin et al. and Sweeney et al. has shown that identifiers may be inherent within sequence data. Thus, it may be necessary to begin considering sequencing studies as human subjects research [34].
Conclusion
The views presented so far are the views of ethicists and scientists. What about the patients/donors themselves? What about the public? Hull et al. conducted a survey of 1,193 patients’ attitudes toward the use of anonymous or identifiable data in genetic research. The demographics of these patients are not representative of ordinary people (e.g. 70% female, 80% had surgery). Over 70% of these patients felt that it was moderately/very important to know about prior and ongoing research being done with their sample. When the distinction between anonymous and identifiable samples was made, the reason of desiring to know more about the research shifted from “curiosity” to “confidentiality”. Clearly, patients and donors are conscious of the need to protect their own privacy when data is identifiable. 57% of patients preferred that permission be obtained when data is identifiable. That figure is only 1% higher than those who prefer that permission be obtained when data is anonymous.
Genome privacy will continue to become a more contentious topic as sequencing and genotyping methods become more robust. As the bioethicist Greenbaum warns, if patients/donors do not have the faith that genomic privacy is guaranteed, then these data will be become closed from the public and researchers. Authorized researchers then would be only able to access it. It is important to secure everyone’s genomic privacy to protect the open access/source philosophy of science. Limiting access only to authorized researchers would also harm small lab researchers, who may not have the resources or time to obtain authorization.
The three main tenets of maintaining genomic privacy are informed/continuing consent, control of data access, and improved de-identifying of data. To foster open access of genomic data, it is important to educate patients/donors of the possible risks. It is also important to ensure that there is minimal risk of re-identification of publicly released data.
References
[1] E. S. Lander, “Initial impact of the sequencing of the human genome,” Nature, vol. 470, no. 7333, pp. 187–197, 2011.
[2] P. Muir et al., “The real cost of sequencing: scaling computation to keep pace with data generation.,” Genome Biol., vol. 17, no. 1, p. 53, 2016.
[3] D. Greenbaum, J. Du, and M. Gerstein, “Genomic anonymity: have we already lost it?,” Am. J. Bioeth., vol. 8, no. 911796916, pp. 71–74, 2008.
[4] Wellcome Trust, “Sharing Data from Large-scale Biological Research Projects : A System of Tripartite Responsibility,” Rep. a Meet. Organ. by Wellcome Trust held 14–15 January 2003 Fort Lauderdale, USA, no. January, p. 6, 2003.
[5] Z. Lin, A. B. Owen, and R. B. Altman, “Genomic Research and Human Subject Privacy,” Science (80-. )., vol. 305, no. July, p. 183, 2004.
[6] N. Homer et al., “Resolving individuals contributing trace amounts of DNA to highly complex mixtures using high-density SNP genotyping microarrays,” PLoS Genet., vol. 4, no. 8, 2008.
[7] R. Braun, W. Rowe, C. Schaefer, J. Zhang, and K. Buetow, “Needles in the Haystack: Identifying individuals present in pooled genomic data,” PLoS Genet., vol. 5, no. 10, 2009.
[8] D. Greenbaum, A. Sboner, X. J. Mu, and M. Gerstein, “Genomics and privacy: Implications of the new reality of closed data for the field,” PLoS Comput. Biol., vol. 7, no. 12, 2011.
[9] D. R. Nyholt, C.-E. Yu, and P. M. Visscher, “On Jim Watson’s APOE status: genetic information is hard to hide,” Eur. J. Hum. Genet., vol. 17, no. 2, pp. 147–149, 2009.
[10] S. Sadigh-Eteghad, M. Talebi, and M. Farhoudi, “Association of apolipoprotein E epsilon 4 allele with sporadic late onset Alzheimer’s disease,” Neurosciences, vol. 17, no. 4, pp. 321–326, 2012.
[11] J. M. Butler, “Genetics and genomics of core short tandem repeat loci used in human identity testing,” J. Forensic Sci., vol. 51, no. 2, pp. 253–265, 2006.
[12] A. Edwards, A. Civitello, H. Hammond, and C. Caskey, “DNA typing and genetic mapping with trimeric and tetrameric tandem repeats,” Am. J. Hum. Genet., vol. 49, pp. 746–56, 1991.
[13] A. Edwards, H. Hammond, L. Jin, C. Caskey, and R. Chakraborty, “Genetic variation at five trimeric and tetrameric tandem repeat loci in four human population groups,” Genomics, vol. 12, pp. 241–53, 1992.
[14] B. Sykes and C. Irven, “Surnames and the Y Chromosome,” Am. J. Hum. Genet., vol. 66, no. 4, pp. 1417–1419, 2000.
[15] G. Naik, “Family secrets: An adopted man’s 26-year quest for his father,” Wall Street Journal, 02-May-2009.
[16] J. Lunshof, R. Chadwick, D. Vorhaus, and G. Church, “From genetic privacy to open consent,” Nat. Rev. Genet., vol. 9, pp. 406–411, 2008.
[17] J. Gitschier, “Inferential Genotyping of Y Chromosomes in Latter-Day Saints Founders and Comparison to Utah Samples in the HapMap Project,” Am. J. Hum. Genet., vol. 84, no. 2, pp. 251–258, 2009.
[18] M. Gymrek, A. L. McGuire, D. Golan, E. Halperin, and Y. Erlich, “Identifying personal genomes by surname inference,” Science (80-. )., vol. 339, no. 6117, pp. 321–324, 2013.
[19] W. W. Lowrance and F. S. Collins, “Identifiability in genomic research.,” Science, vol. 317, no. 5838, pp. 600–602, 2007.
[20] B. Danae Lin et al., “Heritability and Genome-Wide Association Studies for Hair Color in a Dutch Twin Family Based Sample,” Genes (Basel), vol. 6, no. 3, pp. 559–576, 2015.
[21] L. Paternoster et al., “Genome-wide Association Study of Three-Dimensional Facial Morphology Identifies a Variant in PAX3 Associated with Nasion Position,” Am. J. Hum. Genet., vol. 90, no. 3, pp. 478–485, 2012.
[22] F. Liu et al., “A Genome-Wide Association Study Identifies Five Loci Influencing Facial Morphology in Europeans,” PLoS Genet., vol. 8, no. 9, 2012.
[23] S. M. Meystre et al., “Automatic de-identification of textual documents in the electronic health record: a review of recent research,” BMC Med. Res. Methodol., vol. 10, no. 1, p. 70, 2010.
[24] B. A. Malin, “An Evaluation of the Current State of Genomic Data Privacy Protection Technology and a Roadmap for the Future,” J. Am. Med. Informatics Assoc., vol. 12, no. 1, pp. 28–34, 2005.
[25] IUPAC, “IUPAC Codes.” [Online]. Available: http://www.bioinformatics.org/sms/iupac.html. [Accessed: 11-Nov-2016].
[26] B. Malin and L. Sweeney, “How (not) to protect genomic data privacy in a distributed network: using trail re-identification to evaluate and design anonymity protection systems,” J. Biomed. Inform., vol. 37, pp. 179–192, 2004.
[27] E. F. Codd, “A Relational Model of Data for Large Shared Data Banks,” Commun. ACM, vol. 13, no. 6, pp. 377–387, 1970.
[28] L. Habegger et al., “RSEQtools: A modular framework to analyze RNA-Seq data using compact, anonymized data summaries,” Bioinformatics, vol. 27, no. 2, pp. 281–283, 2011.
[29] D. Greenbaum and M. Gerstein, “The role of cloud computing in managing the deluge of potentially private genetic data.,” Am. J. Bioeth., vol. 11, no. 11, pp. 39–41, 2011.
[30] N. Eriksson et al., “Web-based, participant-driven studies yield novel genetic associations for common traits,” PLoS Genet., vol. 6, no. 6, pp. 1–20, 2010.
[31] G. Gibson and G. P. Copenhaver, “Consent and internet-enabled human genomics,” PLoS Genet., vol. 6, no. 6, pp. 1–3, 2010.
[32] G. Church et al., “Public access to genome-wide data: Five views on balancing research with privacy and protection,” PLoS Genet., vol. 5, no. 10, pp. 3–6, 2009.
[33] M. A. Austin, S. E. Harding, and C. E. McElroy, “Monitoring ethical, legal, and social issues in developing population genetic databases,” Genet. Med., vol. 5, no. 6, pp. 451–457, 2003.
[34] A. L. Mcguire and R. A. Gibbs, “No Longer De-Identified,” Science (80-. )., vol. 312, pp. 370–371, 2006.